AI Explainability
Designing Local Model-agnostic Explanation Representations
This research project is published and available at ACM Transactions on Interactive Intelligent Systems. The tool has been released as open source and can be downloaded from GitHub.
Project Abstract: In eXplainable Artificial Intelligence (XAI) research, various local model-agnostic methods have been proposed to explain individual predictions to users in order to increase the transparency of the underlying Artificial Intelligence (AI) systems. However, the user perspective has received less attention in XAI research, leading to a (1) lack of involvement of users in the design process of local model-agnostic explanations representations and (2) a limited understanding of how users visually attend them. Against this backdrop, we refined representations of local explanations from four well-established model-agnostic XAI methods in an iterative design process with users. Moreover, we evaluated the refined explanation representations in a laboratory experiment using eye-tracking technology as well as self-reports and interviews. Our results show that users do not necessarily prefer simple explanations and that their individual characteristics, such as gender and previous experience with AI systems, strongly influence their preferences. In addition, users find that some explanations are only useful in certain scenarios making the selection of an appropriate explanation highly dependent on context. With our work, we contribute to ongoing research to improve transparency in AI.

ANCHORS
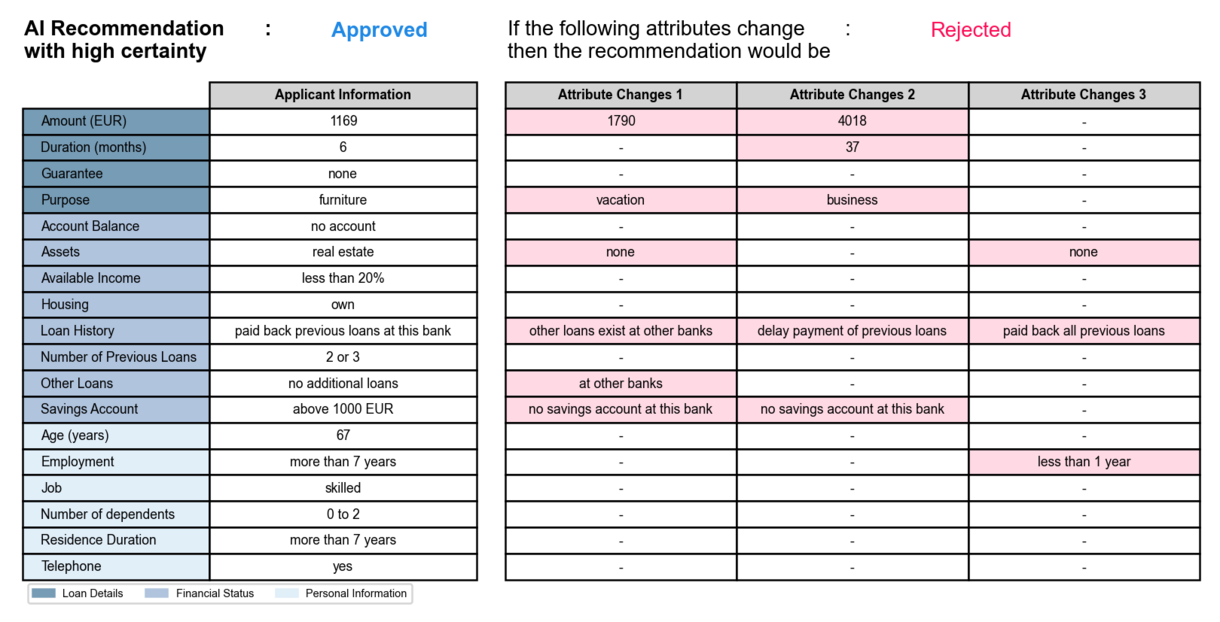
DICE

LIME
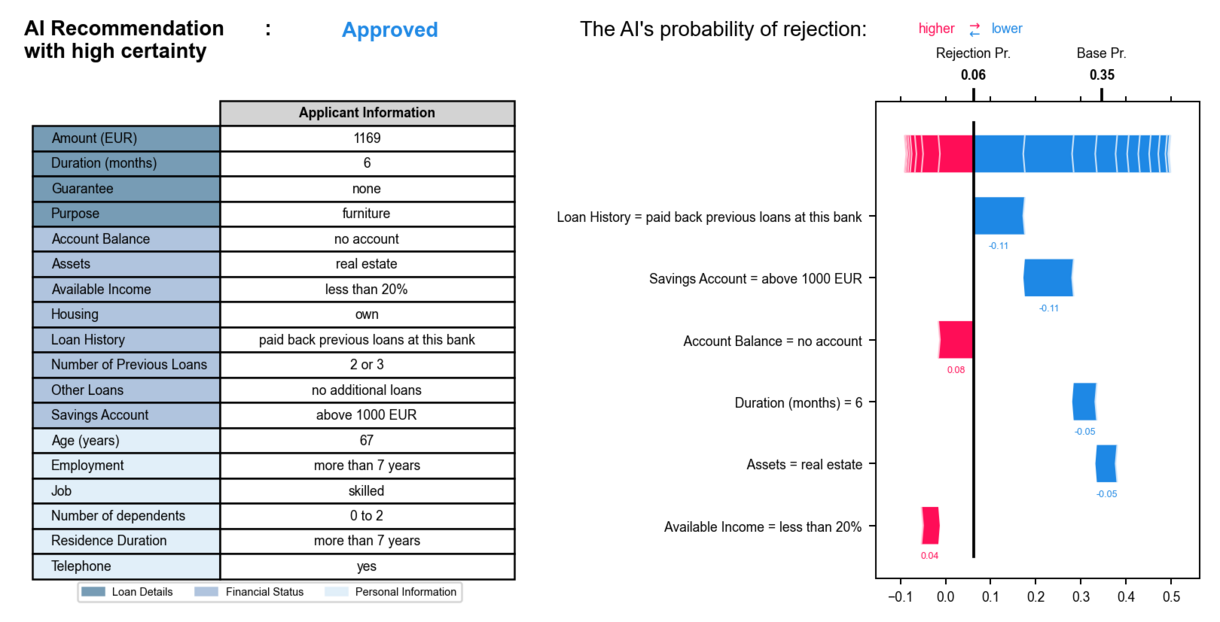
SHAP
This figure shows four explainable artificial intelligence methods in the context of loan applications. The first method, Anchor shows rules that determine the AI system’s recommendation to approve or deny a loan application. If all conditions of these rules are satisfied, that is, that certain attributes’ manifestations are satisfied, then changing the manifestations of other attributes that are not part of the rules cannot change the AI system’s recommendation. The second method, DICE presents three columns. Each column shows how certain manifestations of the loan application’s attributes would have to change in order to reverse the AI system’s recommendation to its opposite, that is, from “Rejected” to “Approved” or vice versa. The third method, LIME shows a bar chart of the six attributes that have the greatest influence on the AI system’s recommendation. The remaining attributes have less influence on the recommendation and are not shown. Here, blue bars indicate an influence on the “Approved” recommendation, while red bars indicate an influence on the “Rejected” recommendation. The values and length of the bars indicate the magnitude of the influence. The final method, SHAP shows how the attributes of the loan application influence the probability of the AI system rejecting the application. The rejection probability is calculated by adding the influence of all attributes to the base probability. The base probability represents the proportion of loan applications that have been rejected in the past. Attributes that increase the rejection probability are represented by red arrows, while attributes that decrease the rejection probability are represented by blue arrows. The length of the arrows and the values indicate the magnitude of the influence. It should be noted that the AI system considers all attributes when computing the recommendation. However, only the details of the six most influential attributes and their values are shown.